From Data Collection and Management to
Activation for Analysis or Customer Engagement
What is Mobile Data and Why is it Important?
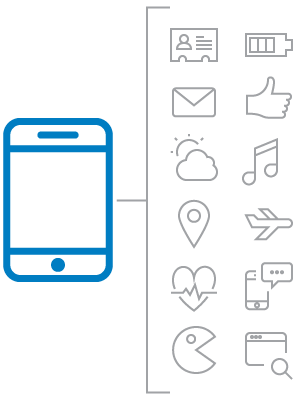
So, what is mobile data? No, we’re not talking about the data that comes with your cell phone subscription plan. We’re talking about the vast amounts of data that your customers are generating while engaging on their mobile devices. This data might come from a website browsed on a mobile phone, a native mobile app, or even the mobile device itself (for example, GPS coordinates), among a multitude of mobile data sources.
Mobile data is unique and complex (check out our glossary of mobile terminology), yet to leverage it most effectively organizations need to integrate this data with all other customer data or risk creating inconsistent and potentially irrelevant customer experiences due to incomplete insights.
With content consumption moving mainly to mobile from desktop computers (and more and more data coming from connected devices and IoT), it’s more important than ever for organizations to unify their data strategy to include a vast array of new types of data. Don’t fall into the trap of accidentally creating yet another silo of data by managing mobile as if it’s merely another channel though. Bring these new data types into a comprehensive, unified data supply chain that can function as a foundation for providing a consistent, relevant customer experience across all venues for customer engagement.
Already know the basics? Check out How to Approach Mobile Data Management. If not, read on…
Unique Considerations for Managing Customer Data from Mobile Devices
Managing the data that originates from mobile devices presents a set of challenges unique from historical tracking challenges that brands may be more familiar with on the web. Consider;
- 1. Collecting Data
- 2. App Stores
- 3. Customer Engagement
- 4. Mobile Signal
- 5. Battery Life
Collecting and using native mobile app data is entirely different than collecting and using data from the mobile web. Mobile apps and websites built to accommodate mobile phones can use drastically different technologies and development techniques (for example, Google AMP HTML), calling for radically different ways to collect, manage and deliver the data, whether that data is needed for analysis or to power customer experience. Not only is the data managed differently, but the data collected still needs to integrate with other data sources that aren’t natively compatible. More on this below. Additionally, some data from mobile has an entirely different taxonomy than the web. Consider the measurement of pageviews on a website versus measuring the ‘screens’ that a user views in a mobile app. Because of how the app is built, ‘screens’ potentially can’t be measured as a ‘pageview’ without taking extraordinary steps to reconcile that data.
Changes to mobile apps require re-submitting them to app stores and an update by users. Unlike websites that can be updated anytime without approvals needed, modifications to mobile apps (even changes to the underlying code) must be re-submitted for approval. Additionally, users must download updates to receive the changes. With the right technology and development processes, apps can be managed strategically to make changes without requiring re-approval or app updates.
Customers engage with brands through various mobile venues and also non-mobile venues. And this will continue for the foreseeable future, with mobile commerce projected to reach 45% of total e-commerce by 2020. You can’t merely buy a mobile-only solution and expect that to solve overarching customer experience challenges that span engagement venues. This approach would cause you to create more problems with siloed data.
Mobile phones generally aren’t as powerful, and may not have as strong of an internet signal, as desktop computers. This means you have to make compromises between tracking, customer experience, and performance. Every personalization requires device resources. Every tracking call needs more. Customers mobile devices only have so much processing power and customers are only willing to wait so long (seconds, maybe).
Mobile phones have limited battery life. This further creates the need to balance performance with customer experience or tracking needs that may drain mobile batteries, just like they consume device processing power.
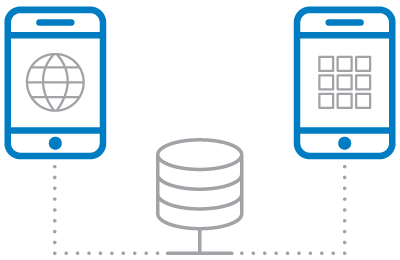
Mobile Data Collection and Delivery
Based on all the mobile-specific considerations and complexities above, managing data from mobile devices requires a host of unique practices, ultimately compelling organizations to take a flexible approach accommodating the diverse ways that customers engage.
What are SDKs? Do I always use SDKs for mobile? How is it different than tagging? Do I even need tags anymore?
Similar to javascript tracking tags, SDKs are collections of code meant to gather and transmit data on user engagement. SDKs are typically utilized in native mobile apps, whereas javascript tags are generally used on websites. However, for all the reasons mentioned above (performance, battery life, varying taxonomies, etc.), managing SDKs requires a different set of strategic compromises than managing javascript tags. Often, there is a tension between delivering the best possible customer experience (fast, relevant) and tracking needs. In short, there’s only so much processing power to go around to drive personalized experiences, report app performance, and track user engagement. SDKs power all of this functionality, but the more SDKs get added, the more performance and customer experience degrades.
This experience degradation can leave developers feeling frustrated that analytics requirements are hampering app performance.. Or marketers may be frustrated because they can’t get the insights needed to make optimizations. This means that companies must adopt technology and processes that accommodate these differences and standardize data between mobile and other sources, whether that data is collected or delivered by an SDK or a javascript tag.
Client-side versus Server-side Data Collection, Management, and Delivery
One way that precious resources can be strategically managed is by utilizing a hybrid strategy that allows for both client-side (on the device) and server-side (in the cloud) management of data. When data can be managed server-side in the cloud, the processing burden moves from the client (the device) to the cloud where there is virtually infinite processing power available. However, managing data via the cloud often can be more expensive, bringing cost considerations into the strategic equation. Ideally, companies adopt technology that gives the flexibility to make this strategic choice balance cost, performance and customer experience.
Can’t I just keep using cookies to get data? What is IDFA?
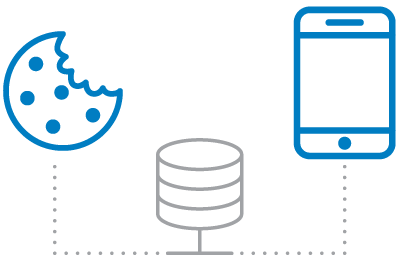
Historically, cookies have been an effective way of managing data client-side. Cookies are downloaded to the client (the device) and can store data on the user’s interactions. However, cookies are increasingly less effective over time as a comprehensive approach to managing data. More and more browsers (both mobile and desktop) and devices are placing restrictions on the use of 3rd party cookies. Where brands used to be able to reliably check a user’s device for a customer ID that may be stored in a cookie, the presence of those cookies are becoming rarer due to customer privacy considerations and increasing government regulations.
To fill this gap in the market, IDFA (‘Identifier for Advertisers’) numbers for Apple devices and AAID (“Android Advertising Identifier”) numbers for Android devices, have been used as a reliable way to identify unique people. So where brands used to be able to check cookies to identify a user, more and more it makes sense to identify users through their IDFA or AAID and then use this or other data as a common identifier to stitch, or integrate, data together that may come from different devices or channels. With the right technology in place, brands can collect IDFA and/or AAID once, then distribute that server-side (if deemed appropriate) instead of relying on the customer device (aka, client) to store that data.
Why and How: Unify Mobile Data into a Comprehensive Data Supply Chain

Why shouldn’t I just pick a new technology to manage mobile data? Isn’t everybody just using mobile phones now anyways?
In short, no. With all the mobile hype out there, it may seem like mobile is the only channel that matters. However, customers judge inconsistent experiences equally harshly whether they happen on mobile, during a support interaction, on the web, or any other venue. Customers don’t exclusively engage on mobile, or only on web, or only in-store, etc. They engage across multiple channels. That’s why it’s so powerful to separate data from execution.
What happens when a customer adds an item to their cart on their mobile device and then purchases the item in store? Well, if the brand hasn’t reconciled and unified mobile data with other data sources, the chances are high the brand will provide an unpleasant customer experience negatively impacting revenue generation. For example, that customer may be retargeted online with advertising for an item he/she already purchased. That’s to say nothing of the positive revenue generation opportunities created for customer engagement by, for example, targeting that customer with cross-selling promotions related to the mobile browsing and in-store data.
OK, so how can I unify mobile data with all other data as a comprehensive data supply chain?
It starts with the capability to collect both mobile and other data sources from a central hub. This means that data collected by javascript tracking tags (whether mobile or other), SDKs, or other methods (for example, ingesting offline data) should ultimately end up in a centralized location.
Then, this brings up the need for all that data, from all those disparate sources with greatly varied formats and taxonomies, to be standardized, reconciled and unified. If this never happens, then the data will remain siloed, which leads to siloed customer experiences that could be irrelevant, or worse, outright inaccurate.
Furthermore this data, that is now standardized and unified, should be actionable in real time. This means that this data, after being transformed at the point of collection, should have the capability to be streamed directly to various destinations to directly power customer experiences or be used for further analysis and insights. This requires the ability to deliver the data client-side and server-side, and the capability to work with both event-level and customer-level data. Ultimately, it’s about flexible technology harnessed by flexible processes built around the customer.
Need More Info or Help? Have Questions?
Tealium’s solution consultants are knowledgeable and ready to help you strategize your unified data strategy incorporating mobile, along all other data sources.